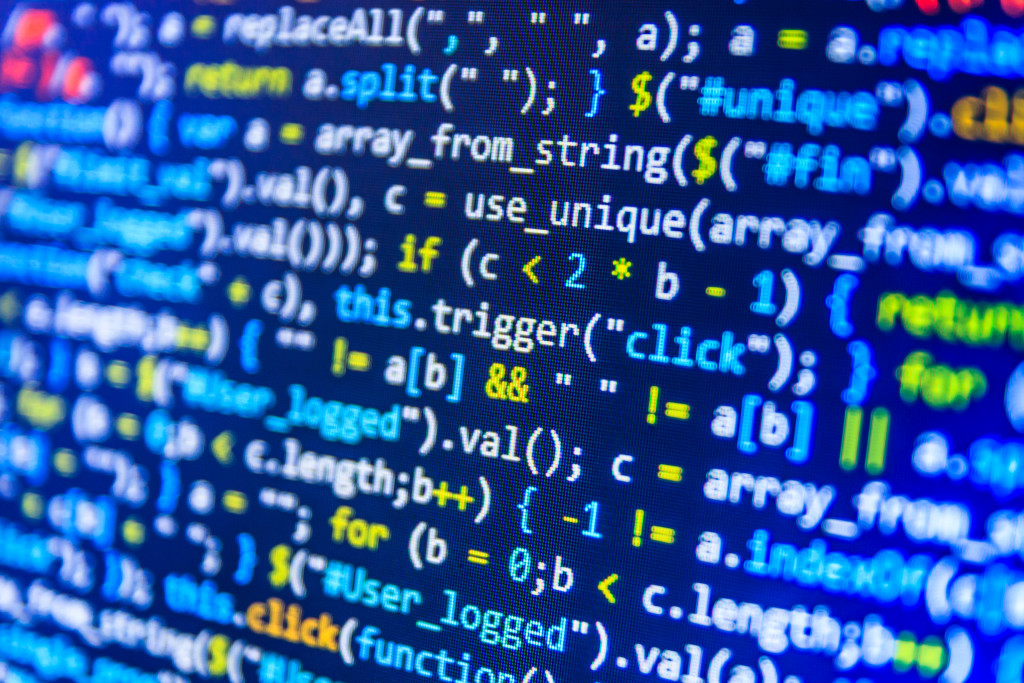
iugo-cafe.org is a project to develop web-based tools for scientific collaboration. Iugo-cafe came about as an experiment to understand the blocks to web-collaboration among scientists and later to experiment with tools that would facilitate code-sharing. The idea was to learn by doing and at the same time to create something that would be of use to our own groups of scientists. The project was led by Eli Holmes in the Mathematical Biology group at the Northwest Fisheries Science Center and supported by NOAA/NMFS.
The project led to the development of three tools:
Chinook
Chinook is a simple content management php toolkit for making interactive websites. It was designed by and for scientists to facilitate on-line workshops. However it is a quite generic tool and could be used many ways. To see a Chinook site being used to provide online statistical workshops, go here Random Pursuits.
Sculpin
Sculpin is a lightweight interface for organizing and distributing software code. It is intended to facilitate code sharing by people who are not computer geeks. It has a simple interface to enable people to create personal pages for sharing code with just a few mouse clicks.
FishBox
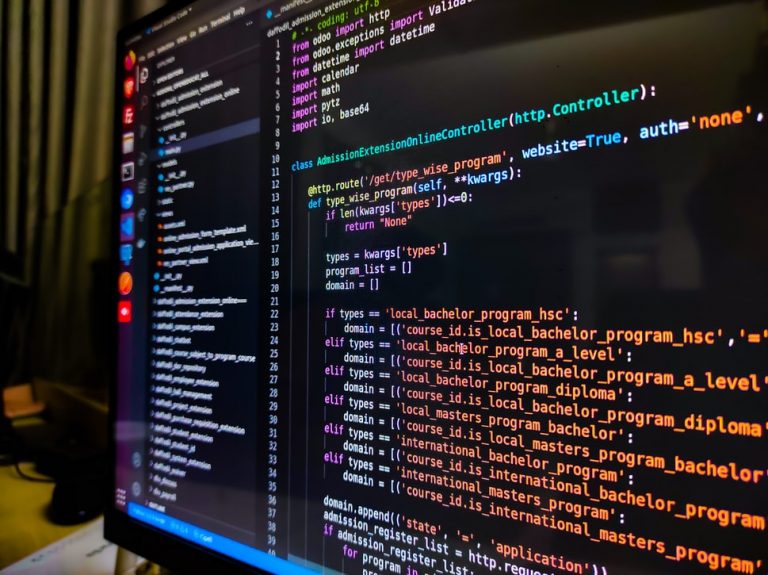
Sculpin is the engine (code) behind FishBox. Fishbox is used by scientists at the Northwest Fisheries Science Center for code-sharing. The site is strong in stochastic processes, population viability, and time-series analysis.
Support
Website maintenance is supported by NOAA/NMFS funding. Development of Chinook and Sculpin were supported by an NWFSC Internal Grant (a competitive program to support innovative pilot projects at NWFSC).